Scientists aren't just working to find a cure for Parkinson's disease, they're also busy finding better ways to catch it early and stifle its development.
Now a new tool that can be run quickly on a standard laptop leverages AI to detect underlying signs of the disease years before symptoms such as tremors and slowed movements start appearing. It's called CRANK-MS: Classification and Ranking Analysis using Neural network generates Knowledge from Mass Spectrometry.
Using trained layers of nodes modeled on the human brain, the tool hunts for specific chemical compounds (metabolites) in the blood, figuring out the patterns that can potentially predict the presence of disease or protect against it.
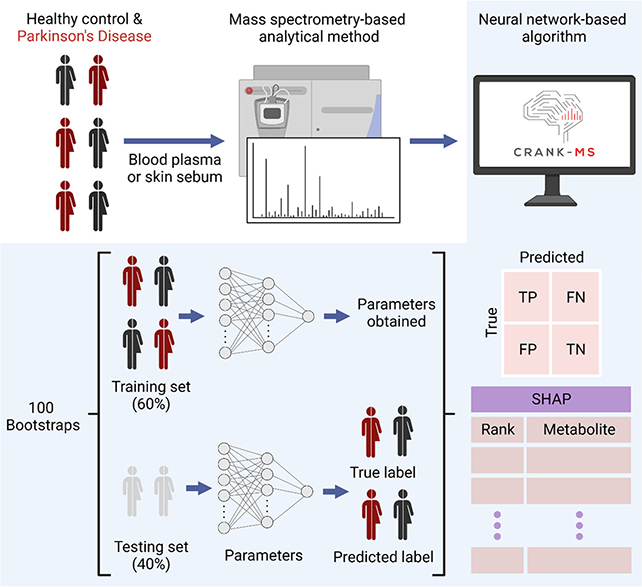
"To figure out which metabolites are more significant for the disease versus control groups, researchers usually look at correlations involving specific molecules," says Diana Zhang, a chemist from the University of New South Wales in Australia.
"But here we take into account that metabolites can have associations with other metabolites – which is where the machine learning comes in. With hundreds to thousands of metabolites, we've used computational power to understand what's going on."
The team made use of blood plasma samples collected as part of the Spanish European Prospective Study on Nutrition and Cancer study. Focusing on 39 patients who went on to develop Parkinson's within 15 years of their participation in the study, the team compared metabolite mixes with 39 control patients who didn't. Several patterns were identified that were considered to be potentially significant.
These metabolites are produced as the body breaks down food, drugs, or chemicals. For example, the team noticed that people who went on to develop Parkinson's tended to have lower levels of triterpenoids in their blood, which handle stress on the body at a cellular level and are found in foods including apples, olives, and tomatoes.
The researchers also noticed the presence of polyfluorinated alkyl substances (PFAS) in people who later developed Parkinson's. That might be linked to higher exposures to industrial chemicals, but larger studies involving much bigger numbers of patients are going to be needed to know for sure.
While this study was a relatively small one, CRANK-MS was able to detect Parkinson's disease risk with an accuracy of up to 96 percent. Part of that was down to the quantity and breadth of data fed into the system right from the beginning, without any need for it to be manually simplified or filtered.
"Here we feed all the information into CRANK-MS without any data reduction right at the start," says chemist William Donald, from the University of New South Wales. "And from that, we can get the model prediction and identify which metabolites are driving the prediction the most, all in one step.
"It means that if there are metabolites which may potentially have been missed using conventional approaches, we can now pick those up."
CRANK-MS is being made available for other scientists to access. That means even more diseases could be detected through blood samples.
The researchers now want to see their system tested on much larger cohorts in more parts of the world to see if the AI analysis holds up for Parkinson's – but the early results are promising in terms of the analysis of metabolites in the blood.
"First, the accuracy is very high for predicting Parkinson's disease in advance of clinical diagnosis," says Donald. "Second, this machine learning approach enabled us to identify chemical markers that are the most important in accurately predicting who will develop Parkinson's disease in the future."
"Third, some of the chemical markers that drive accurate prediction the most have been previously implicated by others to Parkinson's disease in cell-based assays but not in humans."
The research has been published in ACS Central Science.