Nearly two decades after suffering a brainstem stroke at the age of 30 that left her unable to speak, a woman in the US regained the ability to turn her thoughts into words in real time thanks to a new brain-computer interface (BCI) process.
By analyzing her brain activity in 80-millisecond increments and translating it into a synthesized version of her voice, the innovative method by US researchers dispelled a frustrating delay that plagued previous versions of the technology.
Our body's ability to communicate sounds as we think them is a function we often take for granted. Only in rare moments when we're forced to pause for a translator, or hear our speech delayed through a speaker, do we appreciate the speed of our own anatomy.
For individuals whose ability to shape sound has been severed from their brain's speech centers, whether through conditions such as amyotrophic lateral sclerosis or lesions in critical parts of the nervous system, brain implants coupled to specialized software have promised a new lease on life.
A number of BCI speech-translation projects have seen monumental breakthroughs recently, each aiming to whittle away at the time taken to generate speech from thoughts.
Most existing methods require a complete chunk of text to be considered before software can decipher its meaning, which can significantly drag out the seconds between speech initiation and vocalization.
Not only is this unnatural, it can also be frustrating and uncomfortable for those using the system.
"Improving speech synthesis latency and decoding speed is essential for dynamic conversation and fluent communication," the researchers from the University of California in Berkeley and San Francisco write in their published report.
This is "compounded by the fact that speech synthesis requires additional time to play and for the user and listener to comprehend the synthesized audio," explains the team, led by University of California, Berkeley computing engineer Kaylo Littlejohn.
What's more, most existing methods rely on the 'speaker' training the interface by overtly going through the motions of vocalizing. For individuals who are out of practice, or have always had difficulty speaking, providing their decoding software with enough data might be a challenge.
To overcome both of these hurdles, the researchers trained a flexible, deep learning neural network on the 47-year-old participant's sensorimotor cortex activity while she silently 'spoke' 100 unique sentences from a vocabulary of just over 1,000 words.
Littlejohn and colleagues also used an assisted form of communication based on 50 phrases using a smaller set of words.
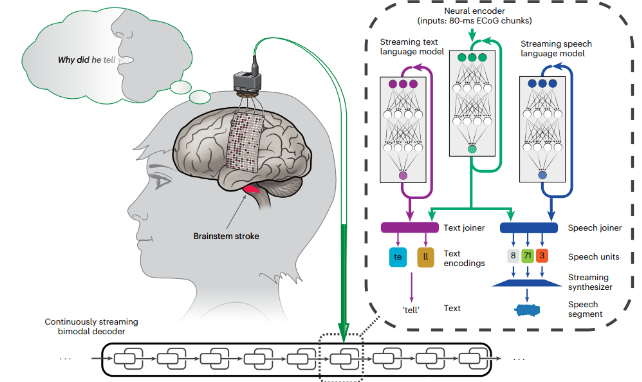
Unlike previous methods, this process did not involve the participant attempting to vocalize – just to think the sentences out in her mind.
The system's decoding of both methods of communication was significant, with the average number of words per minute translated close to double that of previous methods.
Importantly, using a predictive method that could continuously interpret on the fly allowed the participant's speech to flow in a far more natural manner that was 8 times faster than other methods. It even sounded like her own voice, thanks to a voice synthesis program based on prior recordings of her speech.
Running the process offline without limitations on time, the team showed their strategy could even interpret neural signals representing words it hadn't been deliberately trained on.
The authors note there is still much room for improvement before the method could be considered clinically viable. Though the speech was intelligible, it fell well short of methods that decode text.
Considering how far the technology has come in just a few years, however, there is reason to be optimistic that those without a voice could soon be singing the praises of researchers and their mind-reading devices.
This research was published in Nature Neuroscience.